Satellite Precipitation
Error Analysis of Precipitation Estimates
Reasonable estimates of uncertainty are required for proper merging of information from multiple data and model sources, model structure and performance evaluation, ensemble development, and parameter estimation. Uncertainty estimates for the PERSIANN precipitation estimates will be developed via a scaling procedure to enable evaluation of the product against radar data and data from regions where the standard error of ground-based observations is low.
In the semi-arid SWUS, such regions are scarce (especially for unpopulated and remote mountain areas) and, while radar data do give better coverage, they suffer from considerable mountain blockage. We intend to derive scaling relationships that model the uncertainty (standard error) of combined radar/satellite estimates. The figure below shows that the standard errors of PERSIANN estimates in different regions are linearly related to the magnitude of the monthly average precipitation, with the slope of the relationship being related to spatio-temporal resolution. This relationship enables our generalization of PERSIANN the error properties over the regions where gauge and/or radar observations are limited or missing.
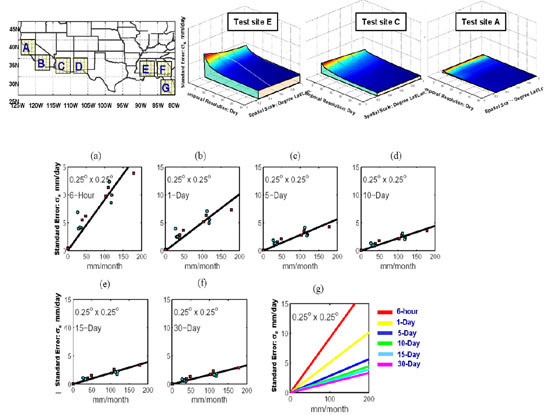
Top panel: similarity in variability of standard errors of PERSIANN estimates in space and time scales (from 6-h, 0.25o x 0.25o to monthly, 1.0o x 1.0o) for seven (A-G) regions, in August 2002. (2) Lower panel: the standard errors of PERSIANN estimates at different temporal resolutions in August 2002 and February 2003 for the seven regions (seven points for each month in each scatterplot) tend to be linearly related to the monthly rainfall amounts of the regions.
PERISANN Validation Links
US (hosted by NOAA-CPC/NCEP)
Australia: Hosted by the Bureau of Meteorology, Australia
PERSIANN Publications
Hsu, K., X. Gao, S. Sorooshian, and H.V. Gupta, “Precipitation Estimation from Remotely Sensed Information Using Artificial Neural Networks,” Journal of Applied Meteorology, Vol. 36, No. 9, 1176-1190, 1997. (pdf file)
Hsu, K., H.V. Gupta, X. Gao, and S. Sorooshian, “Estimation of Physical Variables from Multiple Channel Remotely Sensed Imagery Using a Neural Network: Application to Rainfall Estimation,” Water Resources Research, 35(5), 1605-1618, 1999. (pdf file)
Hsu, K., H.V. Gupta, X. Gao, and S. Sorooshian, “Rainfall Estimation from Satellite Imagery”, Chapter 11 of Artificial Neural Networks in Hydrology, Edited by R.S. Govindaraju and A.R. Rao, Published by Kluwer Academic Publishers, P.O. Box 17, 3300 AA Dordrecht, The Netherlands, pp 209-234, 2000.
Sorooshian, S., K. Hsu, X. Gao, H.V. Gupta, B. Imam, and Dan Braithwaite, “Evaluation of PERSIANN System Satellite-Based Estimates of Tropical Rainfall,” Bulletin of the American Meteorological Society, Vol. 81, No. 9, 2035-2046, 2000. (pdf file)
Hsu, K., H.V. Gupta, X. Gao, S. Sorooshian, and B. Imam, “SOLO–-An Artificial Neural Network Suitable for Hydrologic Modeling and Analysis”, Water Resources Research, Vol.38, No.12. 1302, 2002. (pdf file)
Sorooshian, S., X. Gao, K. Hsu, R.A. Maddox, Y. Hong, B. Imam, and H.V. Gupta, “Diurnal Variability of Tropical Rainfall Retrived from Combined GOES and TRMM Satellite Information,” Journal of Climate, Vol.15, 983-1001, 2002. (pdf file)
Satellite Precipitation Activities:
Other Links:
Outside Resources
NASA Tropical Rainfall Measurement Mission (TRMM)
NASA Global Precipitation Measurement
Global Precipitation Climatology Project (GPCP)
Global Precipitation Climatology Centre (GPCC)
International Precipitation Working Group (IPWG)
NOAA Global IR Data
NOAA NexRAD Radar Data