Hydrologic Prediction
Assimilation of Satellite Snow Data
The problem of how to predict snow water equivalent (SWE) using numerical models still requires considerable attention. EOS satellite-based remotely sensed snow data provide more realistic information about the snow distribution and quantity than the model predictions. In studies involving the SAST model coupled with the NCAR GCM (CCM3), we replaced the modeled snow cover over the Rocky Mountains with satellite SMMR monthly snow coverage and SWE data. Significant consequences in terms of both the land surface and atmospheric processes were observed: (1) previously overestimated soil moisture became much closer to the long-term reanalysis values, (2) runoff in the Missouri River basin decreased, (3) regional surface temperature from March to September increased 1 ° -1.5 ° C and became closer to the observations, and (4) summer (JJA) precipitation in the SWUS and Great Plains improved from original substantial underestimation (Jin, 2002). We intend to follow up on these promising results by investigating the physical processes giving rise to the observed changes and implementing appropriate assimilation techniques using the EOS snow data.
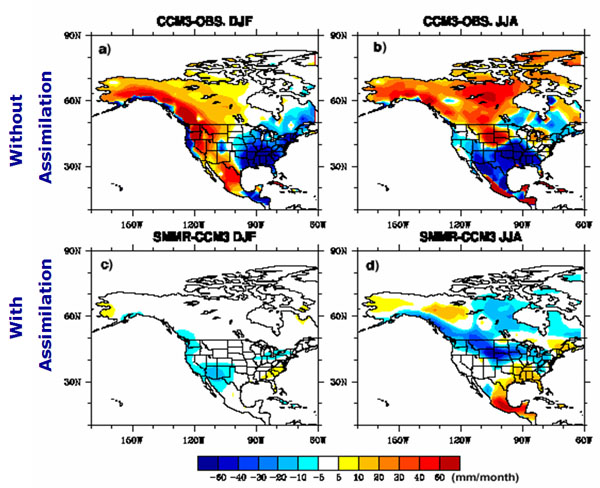
When assimilated into CCM3, the community climate model, MODIS snow information resulted in significant changes of model's prediction of precipitation for long-term simulation.
MODIS snow products are available.
- Improve Mesoscale Modeling
- Improve time-varying boundary conditions using EOS SST data
- Improving the simulated triggering of convective events in urban areas
- Ensemble Weather Forecasting
- Improving snow modeling component in Land Surface Models
- Assimilation of Satellite Snow Data
- Usage of Remote-Sensing data
- Surface Hydrology Forecasting and Prediction System
SWE
Other Links:
National Snow and Ice Data Center
SNOTEL Network
Snow Analysis from NOHRSC